AI Meets the Martian
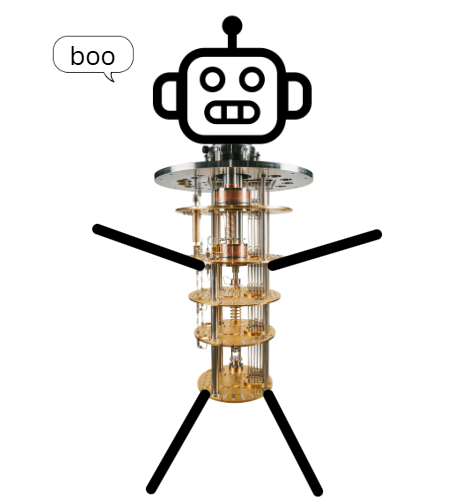
Our two prior quantum blogs, What Even is Quantum?, and then Talking to Elsa, lay the groundwork for where we are picking up today!
We've covered the fascinating quantum system origins, the game-changing rules, and the complexities underlying quantum communication. We've addressed the monumental breakthroughs it poses in the pharmaceutical and cryptographical sectors. We've posed questions of feasibility, and roughly gauged adoption sentiment. But now, we dive deeper into sci-fi's favorite topic: the convergence of AI and quantum technologies. With a solid foundation in superpositioning, entanglement, and a theoretical grasp of the quantum process - we're ready. These make up the diving board we're about to spring off of! In the last installment of the quantum series, we briefly referenced quantum ML as we were discussing functional languages and differentiable capabilities, but left a treasure trove untouched. Today, we're breaking in! The marriage of AI/ML with the Martian could very well alter reality itself.
Well That's Just Classic
The feats AI/ML has already achieved within the classical paradigm have already undeniably revolutionized the world. The bits have rendered us the birth of self-driving cars, the rise of sophisticated chatbots, and the creation of personalized recommendation engines that anticipate our every desire. This remarkable progress is built upon the foundation of classical algorithms like neural networks, support vector machines, and decision trees - all of which are designed to extract meaning from data and make predictions. These algorithms have allowed us to tackle complex problems, from understanding human language to recognizing patterns in images, and even predicting financial trends. Additionally, we've reached the spontaneous generation of multimedia content, as easy as clicking. The blazing success of classical AI as it is, is a testament to the ingenuity of human engineers and the power of the bits to drive progress. AI has gone miles and miles without the Martian, and to major effect - so exactly what does this mystery mouse-ka-tool have to offer the intelligent machine that is currently beyond its grasp?
In achieving these milestones, we've also identified limitations of the classical environment concerning the growth of AI - and these vulnerabilities only continue deepening the further we push on. Like any algorithm in the classical paradigm, AI-powered or not, when performing computations on massive datasets, AI algorithms of even the highest strength are severely strained by the task. This poses frequent bottlenecks, and unclear or truncated insights - because the classical machine is pissed off at you for making it do the one thing it begged you not to: big numbers. Classically-bound AI algorithms have also been known to struggle with issues of overfitting and generalization. Meaning, they struggle with adapting and generalizing to new data when faced with high dimensionality (a multitude of attributes to contextualize) and complex relationships to link throughout the new inputs. This points to the sophistication of the inputs, whereas the prior struggle is regarding sheer scale. And, something we simply accept as fact currently: classical AI models, LLMs in particular, depend entirely upon massive sets of training data - directly antithetical to the PC's liking. The classical paradigm only has so much to offer this burgeoning AI/ML growth, and as the problems we face are exponentially level up in complexity - they demand a quantum leap of us, to once again level the playing field. Accordingly: send in the Martian!
Hop on the Hovercraft!
In addressing these limitations, the Martian's completely foreign mindset stands to break it all open. The inherent nature of classical computation, reliant on bits and linear processing, is no such hurdle in this space. Quantum algorithms can achieve exponential speedup of classical processes - massive and complex datasets? No problem. The superpositioning and entanglement processes we've previously touched on illustrate how this is carried out. This particular point has more or less been made in our work already - but still bares repeating - however, what follows is not old news, so buckle up! In precision, when handling large data, what the classical paradigm is actually offering you, is an extremely close estimation. Close enough to satisfy its queries the vast majority of the time. But - what does the quantum paradigm, likewise, actually render you? The damn answer. Perfect, absolute specificity. In training these quantum ML systems, we're not feeding it a "close enough," succeeded by an endless chain of derivative "close enough"s based on the original estimation - its taking everything its getting down to the tee. This specificity unlocks the door to legitimately perfect insights we have, thus far, considered to be impossible to capture.
The current situation of big data injection dependence is a clearly unideal one, and quantum stands to revolutionize this. The classical AI training system as we know it costs millions, takes months, and upon completion requires heavy doctoring to clean any messes/glaring biases left in its wake. We're shoving a full birthday cake into a human mouth in one go, and cleaning up the mess of what doesn't make it in, or only partially makes it. It's about more Quantum algorithms, offering freedom from procedural ingestion, offer the shift of achieving deep learning through smaller, less structured datasets - similar to the way the qubits disperse and mingle to crack encryptions in Shor's. We could train a model more akin to a gallery of additive united specialists, as opposed to an overwhelming monolith bound to leave crumbs as we feed the machine. Efficiency wouldn't be the only thing to shoot up, reliability would too, and these "specialists" are far more keen to sus out biases in their respective areas. While Classical AI approaches often struggle with nuances and specific domains, quantum algorithms' flexible ability to learn from smaller, more focused datasets tailored to varying focuses - but still achieving total cohesion - presents a massive across-the-board upgrade. This will revolutionize the way we develop language models, leading to more nuanced, context-aware, and efficient applications - that may even materialize in a few moments!
Letting the Qubits Decide
We've referenced quantum ML numerous times, so let's address it. Quantum Machine Learning (QML) presents us with a mysterious potential for enhanced learning, and not just given specificity. The qubits' deep complex interrelationships, impossible in standard bits, are capable of far more magical feats than just knocking down strong encryptions and making medical treatment recommendations in boolean fashion. While Classical Support Vector Machines (SVMs) are powerful tools for classification, they face limitations when dealing with high-dimensional data. Quantum Support Vector Machines (QSVMs) aim to overcome these limitations by leveraging the power of quantum computation. In essence, QSVMs use quantum algorithms to find an optimal hyperplane that separates different classes of data points in a higher-dimensional space. This is achieved through techniques that enhance the efficiency of finding the optimal hyperplane compared to classical SVMs. Especially in analyzing multimedia inputs, like audio and video, the QSVM algorithm has proven highly capable in extracting insights to an extent the classical AI algorithm cannot compete with. There is intuition, there is mysterious wit occurring somewhere in the black box mingling of the qubits, waiting to be harvested. Bridging the gap in understanding we have regarding the nature of qubit interaction within the quantum machine is what unlocks the magic on the other end. By exploiting the principles of superposition, QSVMs can potentially handle a much larger number of features than classical SVMs, making them particularly well-suited for analyzing complex data sets, such as those found in genomics and pharmaceuticals.
To understand the power of quantum computing in drug discovery, let's briefly touch upon the Variational Quantum Eigensolver (VQE) algorithm. VQE is a hybrid quantum-classical algorithm used to find the lowest energy state (ground state) of a quantum system, such as a molecule. Classically, calculating the ground state energy of a molecule becomes computationally intractable as the number of atoms increases. VQE leverages a quantum computer to efficiently evaluate the energy of a molecule given a set of parameters. These parameters define a trial wavefunction, which is a mathematical description of the molecule's quantum state. Now THIS is where we see some real cooking. A classical optimization algorithm iteratively adjusts these parameters, aiming to minimize the energy calculated by the quantum computer. This iterative process, combining the strengths of both classical and quantum computation, allows VQE to efficiently find the ground state energy, providing crucial information about the molecule's stability and behavior, information vital in drug design. In other words - the Martian made friends with your dog and now they know how to play together?! Researchers are actively working on applying VQE to simulate the interactions of molecules with potential drug candidates, paving the way for more efficient and accurate predictions of drug efficacy and toxicity. This mechanic combined with QSVM's effortless handling of dimensions poses potential for the medical field no reasonable person would've thought possible. This coming breakthrough not only promises faster and higher quality patient care, but also a reduction in the substantial financial burden associated with drug development, a universal win-win.
The Snake Shall Do the Charming
We have already explored PennyLane in the prior quantum piece, but now we zoom out a bit and look at the greater Python family - and its profound impact on the development of QML. Python's unparalleled versatility and flexibility have inadvertently rendered it the harbinger of the QML revolution. Though, this success is anything but accidental. It's a direct result of Python's inherent strengths, and the strategic development of quantum computing software. While its versatility and ease of use are undeniable advantages, Python's role extends beyond simple convenience. Key features and design choices within popular quantum computing libraries built on Python make it uniquely suited for the challenges of QML. Qiskit, technically a cousin of the familiar PennyLane, is IBM's quantum Python baby. Qiskit enables its users to create, simulate, and run quantum circuits on IBM's proprietary quantum machines, and has played an equally major role in the advent of QML. Its success stems not only from its user-friendly interface and extensive documentation but also from its sophisticated features designed for quantum algorithm development. Qiskit's integration with classical machine learning libraries within the Python ecosystem is seamless, allowing for the straightforward creation and testing of hybrid quantum-classical algorithms, a massive pull to the seasoned classical Python engineer.
And wouldn't you know it - another cousin. Cirq is Google's own quantum Python baby - and does essentially the same exact thing as Qiskit, just with Google's machines. Likewise, Braket is Amazon's baby... you get the picture. The prevalence of Python in these frameworks underscores its importance not just in classical AI/ML but also in the emerging field of quantum AI/ML. Those aspiring to work in the professional tech space - at all, not just developers - will all speak Python as a requirement to entry soon. The seamless integration of the Python collection libraries for classical machine learning with quantum computing SDKs simplifies the development of hybrid quantum-classical algorithms - a crucial aspect of current QML research. Researchers can readily combine the strengths of classical machine learning techniques with the unique capabilities of quantum algorithms, leading to more powerful and efficient solutions. This ability to easily combine classical and quantum methods is a critical factor driving innovation and adoption in the QML field, largely spearheaded by both the greater Python family, and Q#, which we already covered in Talking to Elsa. The fact that Python acts as a pillar of this integration is a testament to its enduring influence in the ever-evolving landscape of artificial intelligence. The future of QML is bright, and Python’s integral role in that future is undeniable.
Cobi Tadros is a Business Analyst & Azure Certified Administrator with The Training Boss. Cobi possesses his Masters in Business Administration from the University of Central Florida, and his Bachelors in Music from the New England Conservatory of Music. Cobi is certified on Microsoft Power BI and Microsoft SQL Server, with ongoing training on Python and cloud database tools. Cobi is also a passionate, professionally-trained opera singer, and occasionally engages in musical events with the local Orlando community. His passion for writing and the humanities brings an artistic flair with him to all his work! |
Tags:
- AI (3)
- ASP.NET Core (3)
- Azure (13)
- Conference (2)
- Consulting (2)
- cookies (1)
- CreateStudio (5)
- creative (1)
- CRMs (4)
- Data Analytics (3)
- Databricks (1)
- Event (1)
- Fun (1)
- GenerativeAI (4)
- Github (1)
- Markup (1)
- Microsoft (13)
- Microsoft Fabric (2)
- NextJS (1)
- Proven Alliance (1)
- Python (6)
- Sales (5)
- Sitefinity (12)
- Snowflake (1)
- Social Networking (1)
- SQL (2)
- Teams (1)
- Training (2)
- Word Press (1)
- Znode (1)
Playlist for Sitefinity on YouTube
Playlist for Microsoft Fabric on YouTube
Playlist for AI on YouTube
Copyright © 2024 The Training Boss LLC
Developed with Sitefinity 15.1.8321 on ASP.NET 8