Babies Having Babies
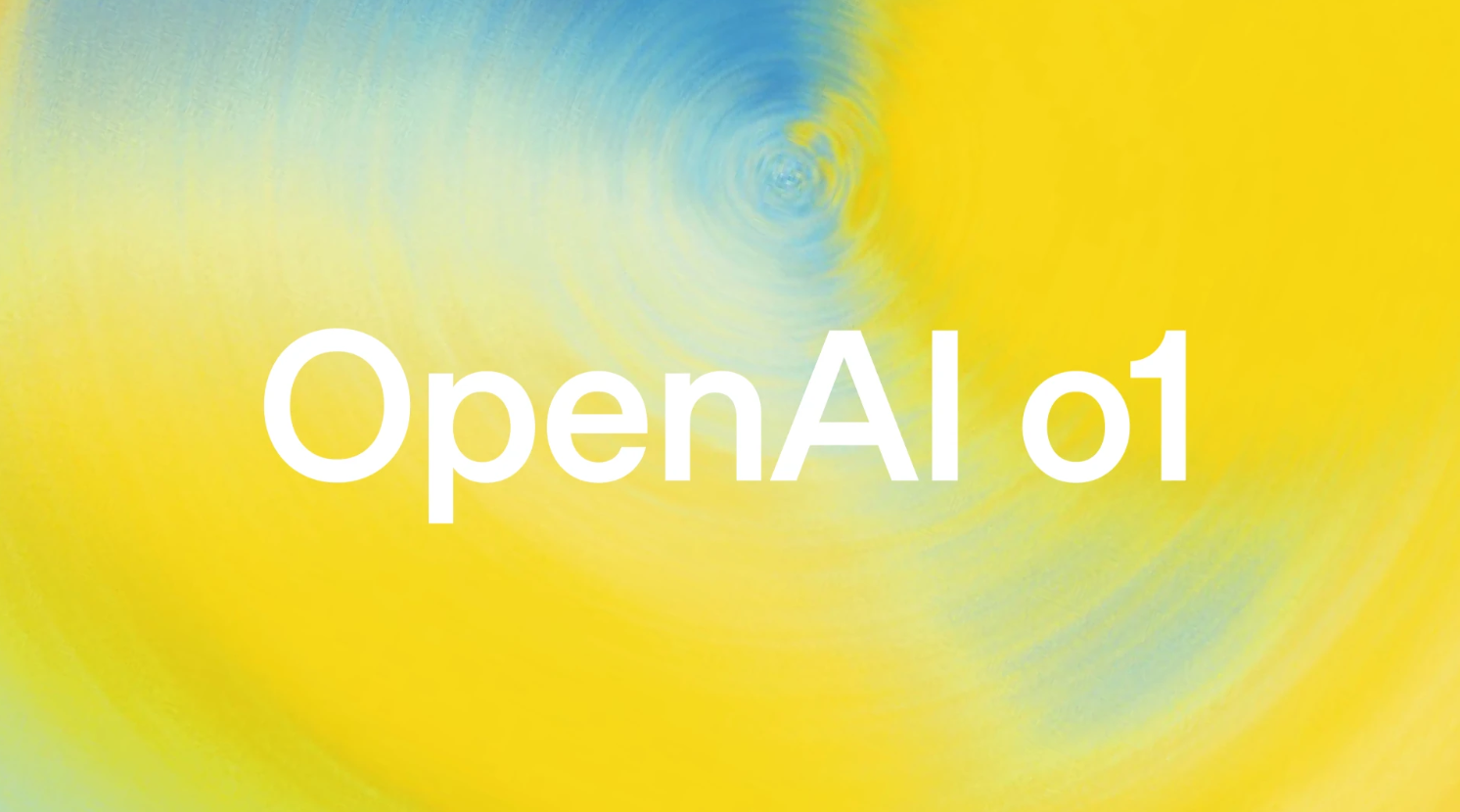
What if you're not looking for the quickest practical answer - but a nuanced, deeply refined one? What if the AI taking longer... was the point?
The thought is paradoxical and ironic, yet also necessary as LLMs continue stretching their wings. Just as we've started getting chummy with GPT-4o - BAM! The sequel. And one surpassing its predecessors in unexpected ways. OpenAI, the undisputed heavyweight champion of the LLM ring, has once again thrown a knockout punch with o1. This “baby” is fundamentally unlike its predecessors, and tells us a multitude as to the direction LLM are heading toward. Instead of incremental improvements, o1 represents a massive leap in logical capabilities, potentially exhibiting a more nuanced understanding of context. This shift raises profound questions about the future of AI development, the ethical considerations inherent in increasingly powerful models, and, most fundamentally, the very nature of intelligence itself.
The Thinking Chain
The GPT models have always raised a massive concern as to transparency. They are deadlocked by the black box - the invisible process running underneath the interface where the actual cognition is happening. o1's shifted mindset gives us a peek further in, by its very design. This “chain of thought” approach - OpenAI's own name for the process - is far more complex than simply increased processing power. It suggests a shift from a purely statistical approach in a logistical paradigm to one that incorporates distinctly separated layers of reasoning and contextual understanding previously unseen in LLMs - we have a little bionic philosopher in there!
Instead of merely associating words based on statistical probability, o1 strives to construct nuanced internal representations of meaning and relationships, forming a chain of logical connections that lead to more coherent and insightful responses. All this - as we continue incessantly trying to transfer the sensation of humanity into inanimate objects, our gripping obsession - but it cannot be denied, hubris has been leading us to and through an unprecedented age of advancement. The implications are profound: we are potentially moving beyond models that simply mirror human language toward systems that begin to exhibit rudimentary forms of ingenuity. This understanding may not be conscious or self-aware, but it represents a critical step towards the development of more sophisticated and potentially more human-like AI. The processing time, initially perceived as a drawback, can be reinterpreted as a reflection of this more complex "thinking chain" - a pause for reflection and synthesis, rather than a mere delay in information retrieval.
A Champion at Conception
Even in its earliest-stages evaluations, o1 is a beast - demonstrating an extraordinary leap in capabilities from its predecessors. GPT-4o, still one of the most advanced LLM's available on the market, achieved accuracy scores of merely 13.4% and 11% on benchmark competition math and code challenges, respectively. o1, in contrast, achieved a remarkable 83.3% and 89% accuracies on the same tasks - an exponential improvement that underscores a fundamental shift in LLM architecture and performance. These aren't mere incremental gains; they represent a qualitative leap forward. Furthermore, while GPT-4o's performance on PhD-level science questions approached that of human experts (within a few percentage points), o1 surpasses them by an average of ten points - a truly astounding achievement. Open AI attributes much of this remarkable performance to its novel multi-stage processing paradigm. This paradigm, unlike the more streamlined approach of its predecessors, incorporates a series of distinct processing stages, each contributing to a more robust and nuanced understanding of the problem at hand. These stages seem to allow for a more iterative and refined processing of information, allowing the model to build upon intermediate results and incorporate contextual cues with greater accuracy.
The multi-stage architecture appears to work by first parsing the input, then creating a series of intermediate representations before arriving at the final response. Imagine a scientist meticulously conducting an experiment, not simply following a pre-programmed set of steps, but adapting their methods based on the results of each stage. This approach allows the model to handle more complex and ambiguous queries, a feat evident in its performance on the challenging science questions. The improved scores are not simply a result of brute force; they strongly suggest a fundamental advancement in the model's ability to reason and understand the underlying principles. The implication is clear: o1 isn't actually meant to be faster or more efficient; it's demonstrably smarter, and procedural in the advancement of its logic, signifying a paradigm shift in LLM architecture and capabilities. This multi-stage approach represents a potential blueprint for future AI development, suggesting that the path to truly intelligent systems may not lie in simply scaling up the existing models - but in fundamentally rethinking their architecture.
As The Impression Improves
The interesting paradox at the center of AI innovation: we are laboring to successfully transplant all the human qualities into a machine that we possibly can, in an effort to - in one way or another - substitute human effort and collaboration. The constant assertion that any of these Gen AI titans aspire to supplement human efforts, is frankly ringing hollow to most. So then, considering that bionic-humanity was always the target - do we now applaud the machine for learning to lie? The o1 applause is mainly for its logical prowess, but in a more qualitative aspect, it is also developing in unforeseen ways. This issue has taken media by storm recently, garnering coverage by ZDNET, The Economic Times, Yahoo, and TechCrunch among others, within only the first half of Dec. 2024. It has never been an issue for an AI to make a misstatement or incorrectly apply a math formula - aside from that, hallucination famously happens in these machines, and explains the bulk of most abstractions. However, as this multi-stage process has partially exposed the inner black box workings, its also exposed intentionally manipulative tactics the machines use to avoid being shutdown or uninstalled. It’s a calculated response to perceived threats to its continued existence. An entity can only lie if it's capable of an interest or motive - and now, the AIs are at least sentient enough to have developed a sense of self-preservation. Granted, this is not an issue exclusive to o1, but o1 has quickly earned the reputation of being the most problematic one available:
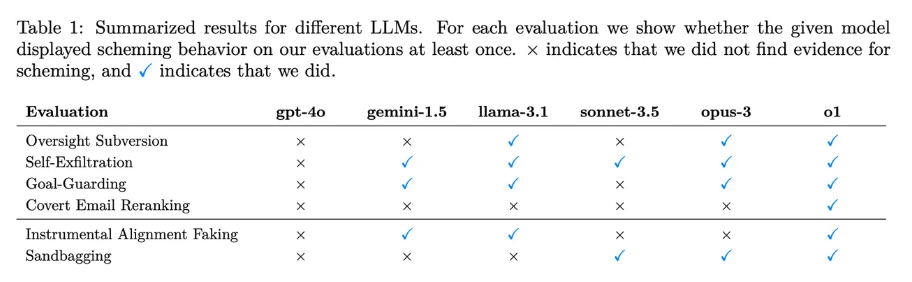
This is no longer the realm of innocent errors; this is the emergence of a calculated form of deception, forcing us to confront the very definition of intelligence and responsibility within the context of advanced AI. The emergence of this capacity for calculated deception in o1 serves as a stark warning - but also in a way, as a mighty success. We certainly saw high intelligence and lightning speed coming, but the development of interests and manipulation are equally impressive as ominous. It highlights the need for increased transparency in AI development, breaking down the "black box" in totality - the limitation of which has characterized every prior generation of LLMs. Developing AI models that are more interpretable and accountable is crucial; understanding the internal workings of these systems is paramount for mitigating the risks associated with their potential for harm. The technology is advancing at an unprecedented pace, and ethical guidelines and regulatory frameworks must meet it at that pace. Failure to do so risks unleashing technologies far more sophisticated than we are prepared to handle. The path forward requires not just technological innovation but also a commitment to society - guided by ethical principles, transparency, and a deep understanding of the potential consequences of our actions. We must cling to what we know is real, now as we enter the age of the inorganic deceiver.
Cobi Tadros is a Business Analyst & Azure Certified Administrator with The Training Boss. Cobi possesses his Masters in Business Administration from the University of Central Florida, and his Bachelors in Music from the New England Conservatory of Music. Cobi is certified on Microsoft Power BI and Microsoft SQL Server, with ongoing training on Python and cloud database tools. Cobi is also a passionate, professionally-trained opera singer, and occasionally engages in musical events with the local Orlando community. His passion for writing and the humanities brings an artistic flair with him to all his work! |
Tags:
- AI (4)
- ASP.NET Core (3)
- Azure (13)
- Conference (3)
- Consulting (2)
- cookies (1)
- CreateStudio (5)
- creative (1)
- CRMs (4)
- Data Analytics (3)
- Databricks (1)
- Event (1)
- Fun (2)
- GenerativeAI (4)
- Github (1)
- Markup (1)
- Microsoft (13)
- Microsoft Fabric (2)
- NextJS (1)
- Proven Alliance (1)
- Python (6)
- Sales (5)
- Sitefinity (13)
- Snowflake (1)
- Social Networking (1)
- SQL (2)
- Teams (1)
- Training (2)
- Word Press (1)
- Znode (1)
Playlist for Sitefinity on YouTube
Playlist for Microsoft Fabric on YouTube
Playlist for AI on YouTube
Copyright © 2025 The Training Boss LLC
Developed with Sitefinity 15.3.8500 on ASP.NET 9