A "Bit" of Prejudice
Machines can only ever be programmed by human individuals - who've inescapably developed perspective. At the very least, perspective. We don't summon artificial "consciousness" from the great null canvas out beyond, they are built. Specifically and meticulously. That quite justly explains why today, the age of AI-mania, society at large is anxious about not just what these machines are being taught - but more importantly, how they're being taught to think. The algorithms powering our increasingly automated world don't merely risk reflecting existing societal biases - they could be used as instruments to amplify them. It is not difficult to build bots that perpetuate inequality and injustice, but the issue of implanted bias goes beyond merely the concern of "bad actors" - as even the well-intentioned could inadvertently corrupt an AI's judgement. From hiring processes that unfairly favor men, to loan applications unfairly denied to minority groups, the consequences are tangible, deeply troubling, and even currently in motion. This isn't a hypothetical threat, it's a current reality.
Data at the Core
The old adage "garbage in, garbage out" soars to new heights in the AI era. The "garbage out" can quickly become noisy, saturated, and increasingly skewed when this electric thought mill is given a disingenuous canvas to paint on from the beginning. Historical biases, issues of representation, and concerns of sampling practices are things the intelligent machine has no way of mitigating for itself - and we need not embrace abstractions to envision the consequences, they've been occurring. The Correctional Offender Management Profiling for Alternative Sanctions (COMPAS) system, a machine learning algorithm developed by Northpointe, uses historical data to predict the likelihood of a priorly convicted individual reoffending. This software has been subject to widespread scrutiny, and was the subject of the 2016 ProPublica article, How We Analyzed the COMPAS Recidivism Algorithm (Larson et al.).
The algorithm, applied across numerous jurisdictions including Florida, Wisconsin, New York, and California, reveals troubling shortcomings. While its overall recidivism predictions boasted approximately 61% accuracy, its accuracy for predicting violent recidivism plummeted to a mere 20%. Given that violent crimes pose the most immediate threat to society, this low accuracy rate is deeply concerning. Furthermore, while COMPAS predicted roughly the same overall recidivism rate for both Black and white defendants (around 60%), a closer examination of a two-year follow-up period revealed significant bias. Black defendants who did not go on to reoffend were incorrectly flagged as high-risk at a rate nearly double that of white defendants (45% v. 23%). Similarly, white defendants were almost twice as likely to be incorrectly classified as low-risk (48% v. 28%). The disparity becomes even more jarring when considering violent recidivism: Black defendants were a staggering 77% more likely to receive high-risk scores than white defendants - a flagrant prejudice, inextricable from the ultimate 20% confidence. It is important to note that the consequences of said biases are befalling incarcerated people as we speak, despite the algorithm's inherent inaccuracy in predicting violent recidivism. The following graphic represents 6,172 defendants who received varying risk assessments (1 = lowest, 10 = highest), but in the following 2 years did not reoffend:
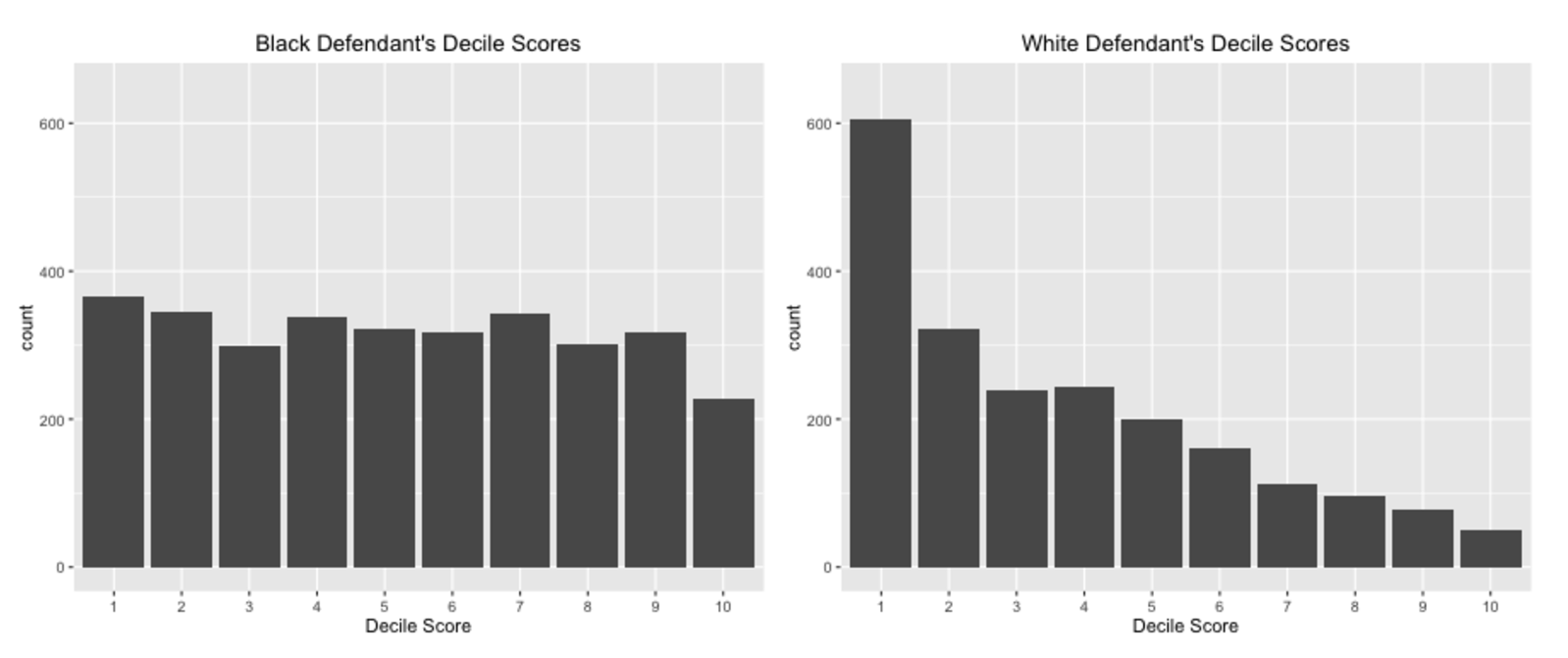
The white defendants' chart looks like a descending staircase with a massive drop off past the lowest-risk score. The black defendants' chart, on the other hand, is nearly equalized across the board. Given that the amalgam has already informed us that any given ex-convict has a 60% of reoffending - it's clear that in truth, COMPAS is semantically skewed to assign black defendants higher risk classifications. This disparity isn't merely a statistical anomaly; it highlights the menacing trifecta of AI bias. First, the ancestry of the data is crucial. The data used to train COMPAS obviously reflects existing racial biases within the American criminal justice system - a system infamously plagued by historical and systemic inequalities. This points to the critical need for rigorous audits of data sources and collection methodologies to ensure representativeness and fairness. Second, this case perfectly illustrates the danger of a feedback loop of bias. If an algorithm disproportionately flags certain groups as high-risk, leading to harsher sentences, that will further skew future data, reinforcing the algorithm's bias. In other words, in its regression COMPAS inadvertently identified blackness as a hefty dummy variable, which could have increasingly adverse effects on black people navigating the legal system in perpetuity. Fundamental to regression, an ignored or irresponsibly-handled variable does not cease to affect the rendered formula, it simply correlates with the error term.
Finally, the COMPAS case underscores the dangerous illusion of objectivity surrounding AI. The algorithm's seemingly objective outputs masked deep-seated biases, lending an unwarranted air of legitimacy to potentially discriminatory decisions. A figure loses any and all alleged "objectivity" when contextualized. These issues demand a critical reassessment of how we develop and deploy AI systems, ensuring transparency, accountability, and a relentless pursuit of equity at every stage. Consider: the black population only comprises 12% of the American population, and simultaneously represent the largest demographic of incarcerated individuals at 32%. Contrariwise, the white population comprises 60% of the country, but is the next largest prison demographic at 31%. There are practically the same amount of white and black people in prison, and this near-parity in incarceration rates, despite vastly different population proportions, reveals that the issue extends beyond race alone. In fact, they most often come from the same communities - showing that this is a macro issue of repeatedly underserved communities.
Yet, society's tendency to over-police and judge the Black community, a bias now embedded in the algorithm itself, is further exacerbated by the disproportionate representation stemming from the smaller Black population, culminating in a fundamentally flawed approach. We're effectively judging a minority by the count of innocents remaining - what did we think we were going to find, something complimentary? Whereas a multitude of socio-economic factors are truly the determinants of this, the algorithm will instinctively correlate race as a noticeable factor - but irresponsibly-trained, will develop to assume causation. The tendency to perceive spurious correlations as evidence of causation has led to grave consequences throughout history - and AI, if left unchecked, risks repeating the same mistakes. This very fallacy led our predecessors to segregate communities in the name of "public health," and not too much earlier, even led them to toss virgins into volcanoes in attempts to make it rain. When we as a species assume a faulty sense of causation, the slope gets steep and extreme quickly. "Objective facts" may appear condemning - but adjustment of perspective can drastically turn an interpretation on its head. How does a machine know that though, how could it?
Correlation, Which is NOT Causality
A thought experiment: you possess a large stack of red solo cups, and a small stack of blue ones. You fill one of each to the brim with vodka - however, you only proceed to chug the blue cup. After you promptly crash out as a result, you come to the conclusion that there is something more inherently dangerous about the color blue to human health. If that sounds incredibly stupid, you read it right! The cup's color is wholly irrelevant, it was the alcohol within responsible for your inebriation. While this analogy is admittedly quite silly to the human mind, a machine doesn't inherently arrive at such rationalizations - an imperative point to remember. Likewise, the race and/or color of an individual who is incarcerated is just that - their race/color - not a indicator of ongoing criminality. But - the anthropological and moral intuitions of the human mind, which easily assert that for us, are nowhere to be found in the hardware. Accordingly, AI systems trained on biased data can easily draw such spurious correlations, problematically linking race or other demographic factors to outcomes like recidivism, as we discussed, but also on broad society-encompassing matters like loan defaults and hiring processes. The vast majority of problematic AIs are not due to malevolence - but face hurdles stemming from the inherent limitations of data analysis, and the wide-open potential for algorithms to misinterpret complex relationships. AI systems excel at identifying correlations - statistical relationships between variables - but can in no way speak to causation - the why behind those relationships. This distinction is critical. To reference Predictive Policing: Review of Benefits and Drawbacks (Meijer & Wessels):
Most of the predictive models are mainly data-driven instead of theory-driven, which can also have major implications on how these models are used. The usage of big data and data-based approaches might have the consequence that there is too much emphasis on correlations, instead of causality (Andrejevic, 2017).
Evidently, AI-driven predictive policing systems often reveal a correlation between crime rates and neighborhood demographics. However, this correlation frequently masks the underlying causes: socioeconomic factors, historical injustices, and biased policing practices. An algorithm, unable to disentangle these complex factors, might wrongly conclude that a specific demographic causes higher crime rates. This leads to increased police surveillance in those communities, creating a self-fulfilling prophecy that reinforces existing inequalities and escalates tensions between law enforcement and marginalized communities. The failure isn't intentional bias but rather a fundamental misunderstanding of correlation versus causation. Even strong correlations, in the absence of causal understanding, can lead to deeply flawed and harmful conclusions, as discussed. This deficiency has profound real-world consequences for community safety and trust in law enforcement. Furthermore, in Discriminatory Discretion... (Davenport, 2023), we are offered a fascinating further look at algorithms' role in the evolving criminal justice landscape:
Davenport's research on pretrial detention algorithms provides a fascinating illustration of algorithmic bias at play within the criminal justice system. The study reveals a curious pattern: police officers consulted the algorithm more frequently for white individuals in misdemeanor cases and more frequently for Black individuals in felony cases. The algorithm, designed to assess the likelihood of culpability and inform warrant decisions, ultimately became a tool reinforcing existing biases. The graph above illustrates how, across every level of severity, black people are issued more warrants for the same crimes. Arguably, the data shows us more than just that - it was used to justify pre-existing biases. Law enforcement sought further information on white individuals suspected of misdemeanors - "hearing them out," per se - but cavalierly delegated the fates of black individuals (standing accused of far more serious charges) to the algorithm's assessments. So, in other words: the police referenced more misdemeanor advice for white people to understand their actions, and referenced more felony advice for black people out of apathy. Systemically, culturally, the literal evidence above - make a pretty strong case for such a claim. This is to say - an intelligent algorithm is an invaluable tool - but it's also very much what you make of it. The machine has circuits, not intentions.
In Our Image
As we've explored these tangible, technical aspects of AI bias and fairness - flawed data, correlation misinterpretation, the resulting mentality of the machine - we must distinguish that the root issues at play are not technical. They are fundamentally social. It is, in fact, arguably the AI's job to reflect and perpetuate the biases, inequalities, and power dynamics inherent in the societies that create them. We do, after all, task it with mimicking human behavior. AI is, in essence, a mirror reflecting our own values: both good and bad. On Halloween 2024, the University of Washington released research unveiling that at least 99% of modern businesses have automated at least some part of their hiring processes. We're far past the discussion of whether or not these algorithms ought to be applied, we're already in the center of the mosh pit. Their research included over 550 real resumes from the white and black, male and female, demographics. "White names" - regardless of an applicant's actual race - were favored 85% of the time, and males were preferred 52% of the time. They also identified that applicants who disclosed a disability were less likely to be marked as a top choice, despite qualification over non-disabled competition. Yet in their holistic study, one point stood out most crucially:
“We found this really unique harm against Black men that wasn’t necessarily visible from just looking at race or gender in isolation,” Wilson said. “Intersectionality is a protected attribute only in California right now, but looking at multidimensional combinations of identities is incredibly important to ensure the fairness of an AI system. If it’s not fair, we need to document that so it can be improved upon.”
Any of these individual issues is alarming, but their work stresses that when multiple of these minority identities intersect, it can have multiplicatively negative repercussions on the applicant. Ironically enough, though, the American public at large has far more faith in these algorithms than human peers, still. The Pew Research Center revealed that 47% of pollsters were in support, whereas only 15% were in dissent of their application. This is symptomatic of society at large assuming AI to be safely removed and objective, despite evidence of the opposite already peeking through. It really speaks volumes to how little the average American trusts their neighbor today - in that they'd overwhelmingly still rather play Russian roulette with a possibly racist robot than trust a stranger with the ability to empathize. The relevance of an algorithm in the arena of loan applications and credit assessment is dead in the water from mention. Credit scores are infamously such bad indicators that the MIT Tech Review recognizes them as not just biased but "noisy" data: synonymous with plagued to the point of uselessness. There is no amount of tech refinement that will make algorithmic loan application processing appropriate in the given paradigm.
We stand at a precipice. The very systems we build to automate and optimize our world simultaneously hold the power to perpetuate and amplify the biases plaguing our society. It is however a double-edged sword, as the positive potential is equally heavy. AI algorithms also are invaluable tools, which, used right, could be used as instruments of equality and opportunity. The fallacy of objectivity surrounding AI must be corrected, we must understand it is a mirror - not an oracle. If we fail to confront the societal injustices reflected within our data, thus relayed to our algorithms, we risk creating a future where technology only exacerbates inequality further. The choice is ours: will we build AI systems that amplify injustice or forge a path toward a more equitable future? The answer determines not merely the future of technology, but the future of community.
Cobi Tadros is a Business Analyst & Azure Certified Administrator with The Training Boss. Cobi possesses his Masters in Business Administration from the University of Central Florida, and his Bachelors in Music from the New England Conservatory of Music. Cobi is certified on Microsoft Power BI and Microsoft SQL Server, with ongoing training on Python and cloud database tools. Cobi is also a passionate, professionally-trained opera singer, and occasionally engages in musical events with the local Orlando community. His passion for writing and the humanities brings an artistic flair with him to all his work! |
Tags:
- AI (4)
- ASP.NET Core (3)
- Azure (13)
- Conference (3)
- Consulting (2)
- cookies (1)
- CreateStudio (5)
- creative (1)
- CRMs (4)
- Data Analytics (3)
- Databricks (1)
- Event (1)
- Fun (2)
- GenerativeAI (4)
- Github (1)
- Markup (1)
- Microsoft (13)
- Microsoft Fabric (2)
- NextJS (1)
- Proven Alliance (1)
- Python (6)
- Sales (5)
- Sitefinity (13)
- Snowflake (1)
- Social Networking (1)
- SQL (2)
- Teams (1)
- Training (2)
- Word Press (1)
- Znode (1)
Playlist for Sitefinity on YouTube
Playlist for Microsoft Fabric on YouTube
Playlist for AI on YouTube
Copyright © 2025 The Training Boss LLC
Developed with Sitefinity 15.3.8500 on ASP.NET 9